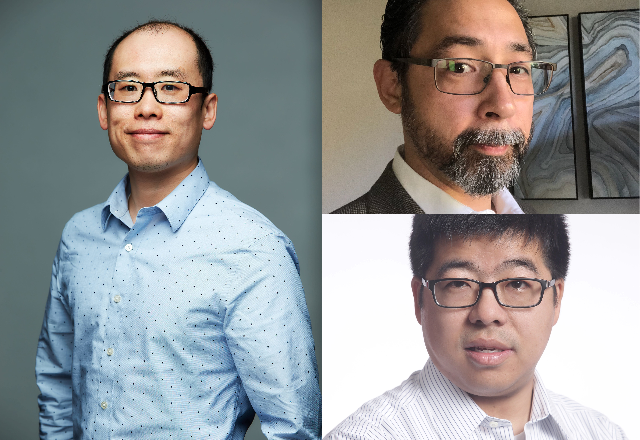
By Lin Chen, Xiang Li, and James Chung who are part of Cambia's AI Team
In the unprecedented circumstance of the global COVID-19 pandemic, Cambia has mobilized to serve people and families. The Data Science and Artificial Intelligence (AI) team supports Cambia’s response by supplying case managers and customer service representatives within the company’s regional health plans with data, analytics, and insights. Figure 1 shows an overview of the usage of data and AI for the COVID-19 response:
- Providing timely testing data
- Generating population-based insights
- Building machine learning models to produce COVID-19 Vulnerability Index
- Tracking COVID-19 symptoms in customer service call notes
- Predicting county-level COVID-19 risk
- Exposing insights through member identification channel to people who work with our members directly
New Data Source
Various existing data sources were utilized for the health plan’s response. This included daily COVID-19 testing results from two major laboratory network providers and tagging those who tested positive from a county’s health care system.
This is a unique data source to triage clinical outreach since it clearly tells if a test is positive or negative and the delay once the test is performed is only in days. Comparatively, our other data sources, such as health insurance claims, have much longer delays -- usually weeks -- and only show that a test is performed. With our data, we can prioritize outreach to members of our regional health plans who recently tested positive much sooner, and we can safely deprioritize health plan members who tested negative.
Artificial Intelligence
We applied AI and machine learning techniques to help Cambia identify, respond to, and reduce the impact of COVID-19.
COVID-19 Vulnerability Index
We used a Machine Learning Model to generate a COVID-19 Vulnerability Index (CVI) that predicts the risk of being admitted to the hospital due to respiratory issues, based on the person’s demographic information and clinical history. Starting with an open sourced model, we validate the model with our own membership population, then build our own model. The model was put into production to generate predications for all members daily, helping multiple business and clinical teams to identify at-risk people and prioritize outreach and resources.
Symptom Tracker
When people call customer service to seek care and information, we apply Natural Language Processing to identify high-risk people and track COVID-19-related symptoms in our call notes data. To stratify health plan members, a scoring system was developed to estimate the urgency of the cases, mainly based on the quantity and severity of the symptoms described in the call notes. The identified member list was validated against nurse notes and the case management queue history. The approach can identify members who might need help due to COVID-19 much sooner than our other identification methods.
Geographical Risk Prediction
Aside from understanding the risk at the individual level, we also wanted to understand the risk at a population level. We built a predictive model to create a single risk index score of COVID-19 on a county-level, capturing both spread and severity. Figure 2 shows the nationwide map of predicted risk scores. The dark red shows higher risk, while the light red indicates lower risk. We can see the model result matches very well with the true COVID-19 cases. Figure 3 incorporated the COVID-19 test results from our membership population in the same map, where the red points represent the positive testing results, while the blue ones are the negative results. We can see the significant matches between the test results and our model predictions.
Member Identification and Smart Queues
The smart queue system we shared in the previous article “Designing Data Systems for Humans: A Case Study in Clinical Identification and Stratification” serves as the channel to surface our solutions to our first-line responders. In addition to queues based on cost, utilization, conditions, and other events, we developed new member identification methods to identify COVID-19 impacted populations. Not only were people at higher risk identified, we augmented the existing identified people with additional COVID-19-related information with the lab data, and our machine model predictions. With this model we can help our first-line responders prioritize the workload and help people in need.
To summarize, we have seen tremendous value of applying data, analytics, and AI to help people in need during this pandemic time. At Cambia, we look forward to innovating more to serve our consumers better with AI.